[pac_divi_table_of_contents included_headings="off|on|on|off|off|off" scroll_speed="8500ms" level_markers_3="none" title_container_bg_color="#004274" _builder_version="4.22.2" _module_preset="default" vertical_offset_tablet="0" horizontal_offset_tablet="0"...
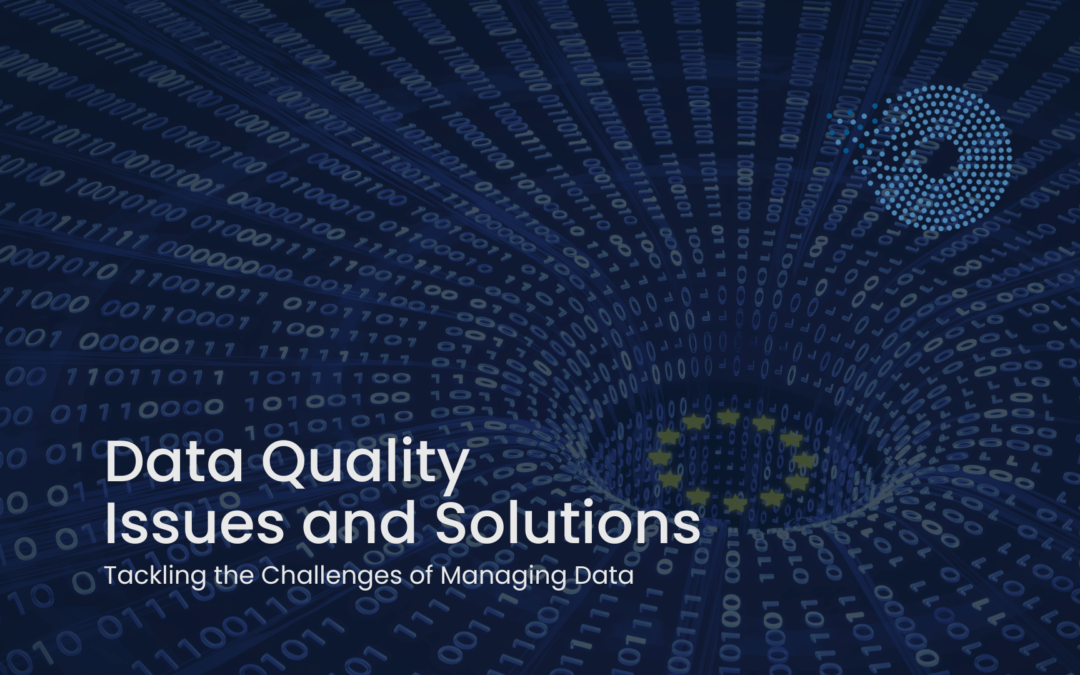
Data Quality Issues and Solutions: Tackling the Challenges of Managing Data
As the world becomes increasingly data-driven, the importance of data quality cannot be overstated. High-quality data is critical to making accurate business decisions, developing effective marketing strategies, and providing high-quality customer service. However, data quality issues can significantly impact the accuracy of analyses and the effectiveness of decision-making. In this article, we’ll explore common data quality issues and how to tackle them through effective solutions and data quality checks.
Data-driven organizations depend on modern technologies and AI for their data assets. However, for them, the struggle with data quality is not unusual. Discrepancies, incompleteness, inaccuracy, security issues, and hidden data are only a few of the long list. Problems associated with data quality can cost companies a small fortune if not spotted and addressed.
Some common examples of poor data quality include…
- Wrong spelling of customer names.
- Incompleteness or obsolete information of geographic locations.
- Obsolete or incorrect contact details.
Data quality directly influences organizational efforts leading to additional rework and delay. Poor quality data practices have a list of disadvantages like it undermines digital initiatives, weakening competitive standing, and directly affecting customer trust.
Some common data quality issues
Poor quality of data is the prime enemy of machine learning used effectively. To make technologies like machine learning work data quality is a must on which we should pay attention. Let’s discuss what are the most common data quality issues and how they can be tackled.
1. Duplication of Data
Due to a massive influx of data from multiple sources such as local databases, cloud data lakes, streaming data, and the application and large system silos. This leads to a lot of duplication and overlaps in these sources. For instance, duplication of contact details ends up contacting the same customer multiple times. That can irritate the customer which can negatively affect the customer experience. On the other hand, some prospects are missed out as well. This can distort the results of data analytics.
Mitigation: Rule-based data quality management can be applied to keep a check on duplication and overlapping of records. We can define predictive DQ rules that learn from the data itself, are auto-generated, and improve continuously. Predictive DQ identifies fuzzy and identical data and quantifies it into a likelihood score for duplicate records.
2. Inaccuracy in data
Data accuracy is vital in the industries like healthcare which are highly regulated. Inaccuracies prevent us from getting a correct picture and planning appropriate actions. Inaccurate customer data can disappoint a customer in personalized customer experiences.
A number of factors such as human errors, data drift, and data decay lead to inaccuracies of data. According to Gartner, 3% of worldwide data gets decayed every month. It causes data quality degradation and compromises data integrity. Automating data management can prevent such issues to some extent, but for assured accuracy, we need to employ dedicated data quality tools. Predictive, continuous, and self-service DQ tools can detect data quality issues early in the data lifecycle and also fix them in most cases.
3. Data ambiguity
After having taken every preventive measure to assure error-free data in large databases some errors will always sneak in such as invalid data, redundancy in data, and data transformation errors. It can get overwhelming for high-speed data streaming. Ambiguous column headings, lack of uniform data format, and spelling errors can go undetected. Such issues can cause flaws in reporting and analytics.
To prevent such discrepancy issues predictive DQ tools must be employed which can constantly monitor the data with autogenerated rules, track down issues as they arise, and resolve the ambiguity.
4. Hidden data
Not all the data is used by organizations. Therefore many fields in the database are kept hidden. That creates large unused data silos.
So when the data is transferred or allowed access to new users the data handler may miss giving them access to the hidden fields.
This can deprive the new data user of some information that could be invaluable for their business. That can cause missing out on spotting new opportunities on many internal and external fronts.
An appropriate predictive DQ system can prevent this issue as it has the ability to discover hidden data fields and their correlations.
5. Data inconsistencies
Data from multiple sources is likely to have inconsistencies in the information for the same data field across sources. There can be format discrepancies, unit discrepancies, spelling discrepancies, etc. Sometimes merger exercises of two large data sets can also create discrepancies. It’s vital to address these inconsistencies and reconcile them otherwise builds up a large silo of dead data. As a data-driven organization, you must keep an eye on possible data consistencies all the time.
We need a comprehensive DQ dashboard to automatically profile datasets, and highlight the quality issues whenever there’s a change in the data. And well-defined adaptive rules that self-learn from data and address the inconsistencies at the source, and the data pipelines only allow the trusted data.
7. Intimidating data size
Data size may not be considered a quality issue but actually, it is. Large sizes can cause a lot of confusion when we are looking for relevant data in that pool. According to Forbes, about 80% of the time business users, data analysts, and data scientists go into looking for the right data. In addition, other problems mentioned earlier get more severe in proportion to the volume of data.
In such a scenario when it’s difficult to make sense of the massive volume and variety of data pouring in from all directions, you need an expert such as [link to DataNectar] on your side who can devise a predictive data quality tool that can scale up with the volume of data, create automatic profiling, detect discrepancies, and changes in the schema, and analyze the emerging patterns.
8. Data downtime
Data downtime is a time when data is going through various transitions such as transformation, reorganizations, infrastructure upgrades, and migrations. It’s a particularly vulnerable time as the queries fired during this time may not be able to fetch accurate information. As a result of the database going through drastic changes, many things change and the addresses in the queries may not correspond to the previous data. Such updates and subsequent maintenance take up the significant time for the data managers.
There can be a number of reasons for data downtime. It’s a challenge in itself to tackle it. The complexity and magnitude of data pipelines add to the challenge. Therefore it becomes essential to constantly monitor data downtime and minimize it through automated solutions.
Here comes the role of a trusted data management partner such as [DataNectar] who can minimize the downtime while seamlessly taking care of the operations during the transitions and assure uninterrupted data operations.
9. Unstructured data
When information is not stored in a database or spreadsheet, and the data components can not be located in (a row, or column) manner, it can be called unstructured data. Some examples of unstructured data are descriptive text, and non-text content such as sound, video, picture, geographical, and IoT streaming info.
Even unstructured data can be rather crucial to support logical decision-making. However, managing unstructured data is a challenge in itself for most businesses. According to a survey by Sail Point and Dimensional Research, a staggering 99% of data professionals face challenges in managing unstructured data sets, and about 42% are unaware of the whereabouts of some important organizational information.
This is a challenge that can not be tackled without the help of intensive techniques such as content processing, content connectors, natural language understanding, and query processing language.
How to tackle data quality issues? Solutions:
First, there is no quick-fix solution. Prevention is always better than cure even in this matter. When you realize that your data has turned into a large mess, the rescue operation is not going to be that easy. It should have prevented this from happening and therefore it is rather advisable that you have a data analytics expert like DataNectar on your side before implementing data analytics so that you can employ strategies to address data quality issues at the source.
It should be a priority in the organizational data strategy. The next step is to involve and enable all stakeholders to contribute to data quality as suggested by your data analytics partner.
Employ the most appropriate best tools to improve the quality as well as to unlock the value of data. Incorporate metadata to describe data in the context of who, what, where, why, when, and how.
The data quality tools should deliver continuous data quality at scale. Also, data governance and data catalog should be used to ensure access to relevant high-quality data in a timely manner to all stakeholders.
The data quality Issues are actually opportunities to understand their nature at their root so that we can prevent them from happening in the future. We must leverage data to improve customer experience, uncover innovative opportunities through a shared understanding of data quality, and drive business growth.
The data quality checks
The first Data Quality check is defining the quality metrics. Then identifying the quality issues by conducting tests, and correcting them. Defining the checks at the attribute level can ensure quick testing and resolution.
Data quality checks are an essential step in maintaining high-quality data. These checks can help identify issues with data accuracy, completeness, and consistency.
The recommended data quality checks are…
- Identifying overlaps and/or duplicates to establish the uniqueness of data.
- Identifying and fixing data completeness by checking for missing values, mandatory fields, and null values.
- Checking the format of all data fields for consistency.
- Setting up validity rules by assessing the range of values.
- Checking data recency or the time of the latest updates of data.
- Checking integrity by validating row, column, conformity, and value.
Here are some common data quality checks that organizations can use to improve their data quality:
- Completeness Checks
Completeness checks are designed to ensure that data is complete and contains all the required information. This can involve checking that all fields are filled in and that there are no missing values. - Accuracy Checks
Accuracy checks are designed to ensure that data is accurate and free from errors. This can involve comparing data to external sources or validating data against known benchmarks. - Consistency Checks
Consistency checks are designed to ensure that data is consistent and free from discrepancies. This can involve comparing data across different data sources or validating data against established rules and standards. - Relevance Checks
Relevance checks are designed to ensure that data is relevant and appropriate for its intended use. This can involve validating data against specific criteria, such as customer demographics or product specifications. - Timeliness Checks
Timeliness checks are designed to ensure that data is up-to-date and relevant. This can involve validating data against established timelines or identifying data that is outdated or no longer relevant.
FAQs about data quality
Q.1 Why is data quality important?
Data quality is critical because it impacts the accuracy of analysis and decision-making. Poor data quality can lead to inaccurate insights, flawed decision-making, and missed opportunities.
Q.2 What are some of the most common data quality issues?
Some of the most common data quality issues include incomplete data, inaccurate data, duplicate data, inconsistent data, and outdated data.
Q.3 How can organizations improve their data quality?
Organizations can improve their data quality by developing data quality standards, conducting data audits, automating data management, training employees on data management best practices, using data quality tools, and implementing data governance.
Q.4 What are data quality checks?
Data quality checks are a series of checks that are designed to ensure that data is accurate, complete, consistent, relevant, and timely.
Q.5 How often should data quality checks be conducted?
Data quality checks should be conducted regularly to ensure that data quality is maintained. The frequency of checks will depend on the volume and complexity of the data being managed.
Q.6 What are some of the consequences of poor data quality?
Poor data quality can lead to inaccurate analysis, flawed decision-making, missed opportunities, and damage to an organization’s reputation.
Conducting data quality checks at regular intervals should be mandatory to assure consistent business performance in any business. You should consider a proactive Data Quality tool that can report quality issues in real time and self-discovers the rules that adapt automatically. With automated Data Quality checks, you can rely on your data to drive well-informed and logical business decisions.
You can determine and set up your data quality parameters with the help of your Data Analytics partner and delegate this exercise to them so that you can focus on strategizing for business growth. This once again proves how important it is to have a Data Analytics partner like Data-Nectar who can take this responsibility freeing you from a hassle.
Conclusion
In conclusion, data quality is critical to making accurate business decisions, developing effective marketing strategies, and providing high-quality customer service. However, data quality issues can significantly impact the accuracy of analyses and the effectiveness of decision-making. By developing data quality standards, conducting regular data audits, automating data management, training employees on data management best practices, using data quality tools, and implementing data governance, organizations can tackle data quality issues and ensure that their data is accurate, complete, consistent, relevant, and timely. Regular data quality checks can also help organizations maintain high-quality data and ensure that their analyses and decision-making are based on accurate insights.
Recent Post
Generative AI Services – 7 best trends for Sector-wide AI Transformation
Getting Started with Power BI: Introduction and Key Features
[pac_divi_table_of_contents included_headings="off|on|on|off|off|off" scroll_speed="8500ms" level_markers_3="none" title_container_bg_color="#004274" _builder_version="4.22.2" _module_preset="default" vertical_offset_tablet="0" horizontal_offset_tablet="0"...
Elevating Customer Service: 9 Key Strategies for Exceptional Customer Experience
[pac_divi_table_of_contents included_headings="off|on|on|off|off|off" scroll_speed="8500ms" level_markers_3="none" title_container_bg_color="#004274" _builder_version="4.22.2" _module_preset="default" vertical_offset_tablet="0" horizontal_offset_tablet="0"...