[pac_divi_table_of_contents included_headings="on|on|on|off|off|off" minimum_number_of_headings="6" scroll_speed="8500ms" level_markers_1="decimal" level_markers_3="none" title_container_bg_color="#004274" admin_label="Table Of Contents Maker"...
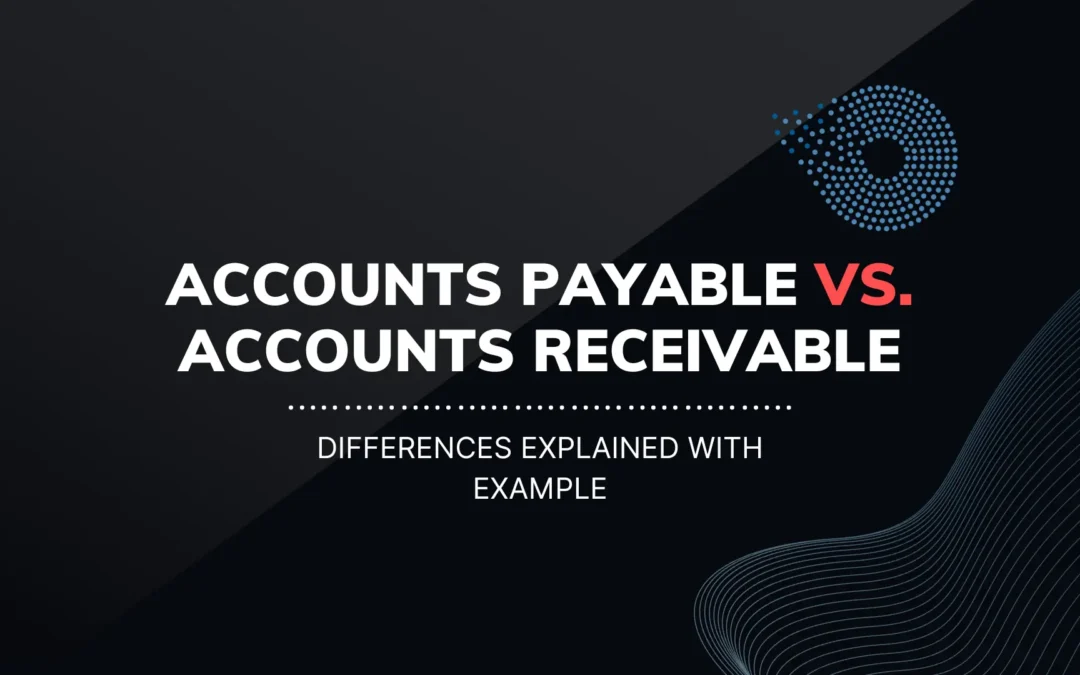
Accounts Payable vs. Accounts Receivable: Differences Explained with Example
Accounts payable and accounts receivable are like the plus and minus signs: When the company’s income and expenses are healthy, it can take advantage of growth opportunities and keep good relationships with clients and suppliers.
Introduction of accounts payable and receivable
In its accounts payable (AP) ledger, a business keeps track of its short-term debts, like buying things from sellers and paying back debts. In business, accounts receivable (AR) are the money the company hopes to get from partners and customers. The accounts receivable definition on the balance sheet is denoted as a current asset.
Lenders and possible investors look at AP and AR to understand how financially stable a business is. It’s essential to make money and spend it wisely so that the company can grow and keep people. Any mistake on either side of the issue can hurt your credit and, in the long run, your business’s stability.
What is accounts payable?
Accounts Payable Understanding: You can keep track of the money you owe any third party with accounts due, a cash flow account. The third parties could be a bank, a business, or even someone you took money from. When you buy something from another company, that’s an example of an accounts payable. The amounts are usually due immediately or within a short time, depending on the terms for return.
Example of accounts payable:
Scenario for XYZ Electronics
XYZ Electronics, a technology company, procures electronic components from various suppliers to manufacture its products. Let’s examine a specific transaction involving Accounts Payable.
Transaction Details:
1) Purchase Order (PO):
XYZ Electronics places a purchase order with Supplier A for electronic components, totaling $10,000.
2) Delivery and Invoice:
Supplier A delivers the components, along with an invoice for $10,000, indicating a payment term of “net 30 days.”
3) Recording the Transaction:
XYZ Electronics receives the components and records the transaction in its accounting system as follows:
Debit: Inventory $10,000
Credit: Accounts Payable $10,000
This entry reflects an increase in inventory (an asset) and the creation of an accounts payable liability.
4) Payment Terms:
The payment terms are “net 30,” meaning XYZ Electronics has 30 days to settle the $10,000 invoice.
5) Payment Processing:
As the due date approaches, XYZ Electronics reviews its payables and initiates the payment process.
6) Payment Execution:
On the 30th day, XYZ Electronics issues a check to Supplier A for $10,000, thereby settling the accounts payable.
Importance and Analysis:
1) Cash Flow Management:
XYZ Electronics effectively manages its cash flow by utilizing the 30-day credit period, ensuring operational funds are available for other needs.
2) Supplier Relationships:
Timely payment strengthens the relationship with Supplier A, fostering reliability and potential future collaboration.
3) Financial Reporting:
The accounting entries provide an accurate snapshot of XYZ Electronics’ financial position, with inventory reflecting the value of components on hand and accounts payable indicating outstanding obligations.
Thus, Accounts Payable is a dynamic component of financial management, balancing cash flow needs with timely payments. This case study illustrates how XYZ Electronics leverages accounts payable to facilitate smooth operations and maintain positive supplier relationships.
What is accounts receivable?
Accounts receivable definition: Customers owe your business money for goods or services that you have billed them for is called accounts receivable. On the balance sheet, the total value of all accounts receivable is shown as current assets. This includes bills that customers owe for goods or work done for them on credit.
Most of the time, vendors bill their clients after providing services or goods based on the terms that both parties agree upon when a contract or purchase order is given.
Customers agree to pay bills within 30 days, called “net 30.” Terms can also be net 60 or even net 90, and a business may agree to them to get a contract. But if you order a lot, especially if the item is made just for you, the company may ask for a deposit upfront. A lot of the time, service companies also charge part of their fees upfront.
When a business gives goods or services to a customer, the accounts receivable (AR) team bills the customer and records the amount owed as an account receivable, making note of the terms.
If the customer pays as planned, the team marks the payment as a deposit. After that, the account is no longer owed money. If the customer doesn’t pay on time, the sales rep or collections team will likely send a “dunning letter.” This letter may include a copy of the original bill and a list of any late fees.
Companies that use accounting and finance software can improve their day’s payables by having customers emailed about invoices that are past due and asking for payment right away.
Accounts that are past due can be drilled down on to get more information about the customer, like:
1. Payment
2. Due date
3. Amount due
4. Credit terms
Keep an eye out for the option to filter out specific customers, such as those on extended terms, from collection emails.
How many types of account receivable are there?
There are three main types of receivables: trade accounts receivable, notes receivable, and other accounts receivable.
1) Accounts Receivable
Credit sales are generally what cause accounts receivable. This scenario happens when you buy things on credit. Most of the time, you have one to two months to make the payment.
2) Notes Receivable
This type of receivable looks like a business letter. The fee for this kind of loan is due in two to three months. If you pay off your debts in that time, you won’t have to pay interest on them. If the debtor asks for more time to pay, however, interest will be added at the rate of one month’s increase.
3) Other receivables
These are different types of receivables, such as interest receivables, wage receivables, employee advances, and tax refunds. Notes are classified as general liabilities on the balance sheet because of their unique characteristics.
Example of accounts receivable
Accounts receivable in a dental practice refer to the outstanding balance of payments owed by patients and insurance companies for dental services rendered but not yet settled.
In this case, the dental bills receivable look like this:
- Patient A has an outstanding balance of $120 for a check-up.
- Patient B has an outstanding balance of $200 for a dental filling.
- The insurance provider of Patient C is responsible for a payment of $600 for a medical service.
- Patient D has a remaining balance of $300 after making a payment of $150.
- The insurance provider for Patient E is responsible for a payment of $170 for a cleaning procedure.
- The deductible for Patient F’s insurance is $80.
The aggregate outstanding payments owed to the company for the month is $1,470. This refers to the funds that you are waiting to get from patients and insurance companies. Effective management is essential for maintaining financial stability.
What Is an Account Receivables Overview Dashboard?
A visual tool called an Accounts Receivable Dashboard is used to manage, evaluate, and keep an eye on activities related to accounts receivable. It gives everyone on the team a clear picture of all the AR statements in one place.
It can also link to other useful tools, like ERP or billing systems. This gives you more information about how customers act and how they pay. It also helps the AR team quickly make sensible decisions when there are customer complaints or late payments.
Metrics for accounts receivable are an important part of your display. You can always see how well your customers pay their bills because the software calculates accounts receivable KPI (key performance indicators for you.
Benefits of an Accounts Receivable KPI Dashboard
Automating A/R includes more than a dashboard. Is it worth the time to add other data or make an AR aging dashboard? It’s worth thinking about the advantages listed below.
#1. Updates in real-time
Companies might not do new Accounts Receivable KPI estimates for weeks or months if they don’t use automation. The A/R team could give these numbers to managers once a month or three times a year so they can look over them. It may seem like something you’d do regularly, but having up-to-date financial data is important for seeing possible issues before they escalate.
#2. Valid Data
The team can stay accurate and up to date on all accounts due data with the help of a dashboard. Workers don’t have to worry about making reports by hand or dealing with spreadsheets that are full of mistakes because of real-time updates.
#3. Details and Insights
The dashboard lets you divide data into parts that are simple to handle. It can help you find patterns, trends, and spots to improve tasks. Managers can compare the different measures to get a better idea of how their team is doing.
#4. Better performance
The AR statement dashboard gives managers real-time information they can use to quickly change strategies or decide which jobs are most important. They can help managers find and fix problems faster if there are changes in how customers act. The following can reduce time wasted on jobs that don’t add value and boost overall performance.
#5. Motivating a Team
The dashboard is also a great way to see how well your team is doing and how well you’re doing as an individual. It helps make things more competitive, which motivates people to do better, and it lets the best workers be recognized. Getting rid of the need to do calculations by hand can also boost team morale.
#6. Better handling of cash
In general, the AR dashboard helps improve on how is accounts receivable a debit or credit. It allows managers to figure out which customers are taking too long to pay, decide how to best collect from them, and make better choices about whether to extend payment terms or do something else.
Final words on accounts receivable KPI
For businesses to get the most out of their A/R dashboards, they need to make sure they choose the right A/R tools. Companies should figure out which specs are most important for running their businesses and keeping their money safe. Also, it should ensure that it works with software and hardware now and in the future.
Lastly, pick a software company you can trust that offers excellent customer service. At Data Nectar, we offer an automatic service that has helped our clients achieve great results. We helped them cut down on DSO and risky debts. Also, our clients have said that they get fewer bills that are past due and faster cash payments. What is Data Nectar going to do for you? To begin, book a demo for the accounts receivable KPI dashboard.
Recent Post
Top Benefits of Data Governance for Your Organization
Enhancing Customer Engagement with Data Management Platforms and Omni-Channel Strategies
[pac_divi_table_of_contents included_headings="on|on|on|off|off|off" minimum_number_of_headings="6" scroll_speed="8500ms" level_markers_1="decimal" level_markers_3="none" title_container_bg_color="#004274" admin_label="Table Of Contents Maker"...
AI Personalization Excellence: Enhancing Customer Engagement in 2024
[pac_divi_table_of_contents included_headings="off|on|on|off|off|off" scroll_speed="8500ms" level_markers_3="none" title_container_bg_color="#004274" _builder_version="4.22.2" _module_preset="default" vertical_offset_tablet="0" horizontal_offset_tablet="0"...