[pac_divi_table_of_contents included_headings="on|on|on|off|off|off" minimum_number_of_headings="6" scroll_speed="8500ms" level_markers_1="decimal" level_markers_3="none" title_container_bg_color="#004274" _builder_version="4.22.2" _module_preset="default"...
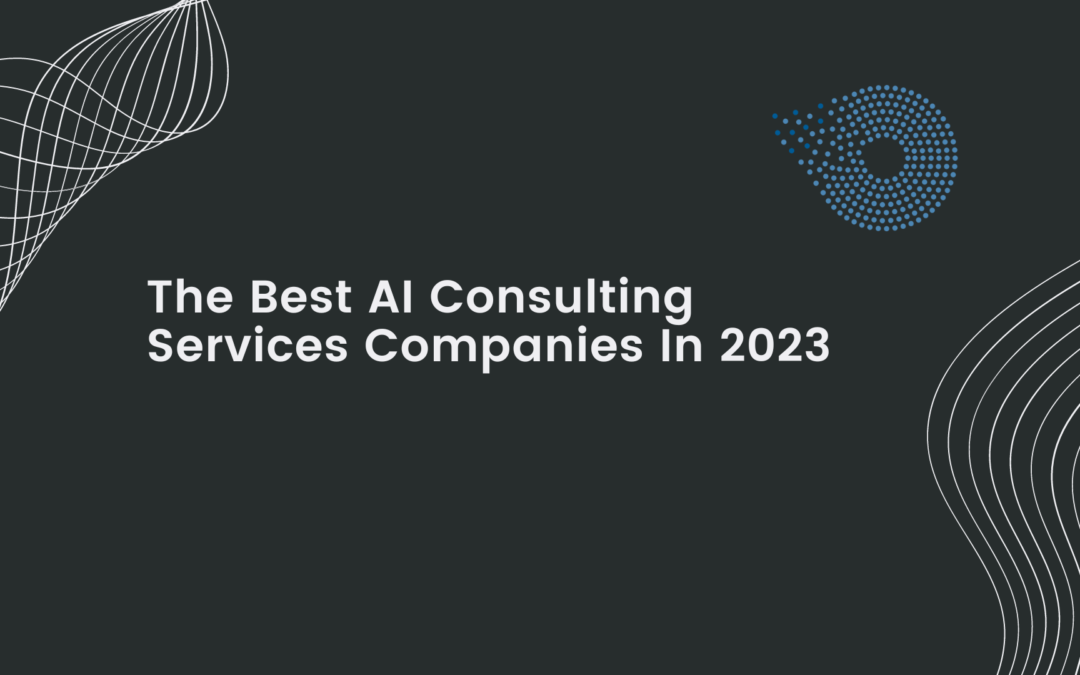
The Best AI Consulting Services Companies In 2023
AI is nearing the next maturity level and coming out of the hype cycle, as per the Gartner report. It is being used in more industries, not just for automation. It is also used to make intelligent products and services for business growth.
Around 90% of businesses in the US and UK see artificial intelligence as important, and most of them have AI projects in the works or the planning stages.
However, half of them admit they lack the expertise to fully leverage AI technologies. Expert AI consulting Services can help in this regard.
What is an AI consulting company?
Consulting firms specializing in AI use this technology to advise and assist businesses.
These are the responsibilities of Expert AI consulting Services employed by these companies:
The best AI technologies and solutions are identified by analyzing the client’s requirements and business goals.
- Creating AI models, algorithms, and programs that meet the customer’s needs.
- Serving as a client’s go-to resource for advice and assistance from planning through launch and beyond.
- Making sure AI systems are safe and follow all applicable rules and regulations.
- Clients will be better equipped to make the most of their AI investments after participating in training sessions and workshops.
- By shedding them, businesses can leverage the most recent AI technologies and expertise to streamline operations, enhance customer experiences, and stimulate growth.
Why are AI data solutions companies important?
Products that can use AI are becoming more important. AI-powered products, especially creative AI-powered products like ChatGPT, are in high demand, but the supply is limited due to a lack of knowledge and resources.
Either the technology isn’t quite there yet, or businesses don’t know which companies to hire. So, Expert AI consulting Services firms are a big part of how businesses and tech companies work together.
The main goal of the best AI development companies is to help people and businesses figure out how to use AI data solutions technology to make their processes better.
What do Expert AI Consulting Services companies do?
A company that does AI consulting gives businesses information and tips about using AI to improve operations. AI Consulting can be broken down into three main parts:
1. Strategy development
To help a business use Artificial Intelligence (AI) successfully, Top AI Development Experts must have a clear understanding of the client’s specific needs, problems, and opportunities. Analyzing the company’s data and analytics capabilities and pinpointing its weak spots are essential steps in this process.
Based on this data, an AI consulting firm can advise a company on the most fruitful AI projects to undertake and how to best integrate and exploit AI to support expansion and success.
2. Implementation
Once an AI strategy is made, it must be put into action through a number of steps and actions. It encompasses all phases of the project life cycle, from initial planning and company selection to project management, implementation, and continuous optimization of business operations. Change management is also very important for making sure that AI is implemented and used well in the company.
3. Education and training
The consulting company should try to improve the client’s information and skills. It is crucial in the competitive field of artificial intelligence. AI consulting businesses mostly help their clients make sure that the people who will be working on the technologies are skilled and knowledgeable about them.
The best AI development companies on our list for 2023
1. Boston Consulting Group
Boston Consulting Group (BCG), a multinational management consulting business, has developed strong AI consulting skills. BCG uses extensive industry expertise and cutting-edge AI technology to create customized solutions for customers.
BCG’s AI consultancy has helped firms improve operations, customer experiences, and innovation through data-driven decision-making.
BCG helps customers make educated decisions with substantial business impact by using powerful AI algorithms and predictive analytics.
BCG’s AI consultancy is distinctive. They value a human-centered approach and address corporate and social ramifications. BCG’s approach covers AI strategy creation, technology implementation, talent management, and ethics.
This complete strategy guarantees that AI solutions meet corporate goals while addressing risks and ethics.
Headquarters: MA, Boston, USA
Founded: 1963
Size: 10000+
2. Data Nectar Technosys
With a global presence in Norway, the UK, India, and the USA, Data Nectar Technosys is committed to helping organizations leverage the power of Data Analytics and Business Intelligence to gain a significant competitive advantage.
Data Nectar Technosys is a dynamic data analytics and consulting services company that collaborates closely with businesses to address complex challenges and deliver data and analytics solutions.
Their team of data scientists and strategists works hand-in-hand with clients to provide high-value solutions in various domains, including Machine Learning, Generative AI, Deep Learning, and Business Intelligence.
They assist their clients in optimizing the utilization of their data assets, enabling them to make informed, timely, and strategic business decisions, whether for the short term or long term.
The company’s core focus is on delivering actionable insights that drive operational improvements and ultimately lead to enhanced profitability for its clients.
Headquarters: UK
Year: 2015
Size: 10- 45
3. Deeper Insights
Deeper Insights is a UK-based AI consulting and development firm that specializes in AI research, ML engineering, NLP, and computer vision. Their website says they tackle “impossible problems” to help clients achieve commercial outcomes.
The company creates unique AI algorithms and ML models utilizing customer data or data from their Skim EngineTM ML web scraper.
They’ve completed a number of cutting-edge AI projects for both large and small clients, a global real estate services company, such as an automated insights app for Deloitte’s sales teams, a custom media monitoring platform for Jll, and a set of deep learning-integrated computer vision algorithms to recognize body parts in images used in robotic surgeries.
Headquarters: London, UK
Year: 2014
Size: 10-49
4. Cambridge Consultants
Cambridge Consultants helps companies create AI-based products that “will change how we live and the world around us.” They have over 20,000 square meters of R&D and development space and a team of 900 people.
Cambridge Consultants, which has been around since 1960 and is part of the French tech consulting company Capgemini, is a big and well-known name on our list of the best AI development companies.
Cambridge Consultants is known for its AI innovations in many businesses and fields. Their projects consist of a drone delivery service to military robots that use AI-based navigation and reinforcement learning.
They also have the technology for spraying crops, an AI system built for the UK Ministry of Defense to automatically respond to cyberattacks, and a tool for classifying piano music.
Headquarters: Cambridge, UK
Year: 1960
Rates: $99+ / hr
Size: 900+
5. Avenga
Avenga is a renowned IT engineering and AI consulting firm that uses cutting-edge innovation to revolutionize businesses. They provide scalable AI solutions to streamline operations, improve consumer experiences, and get insights.
Avenga remains ahead of AI developments by partnering with industry leaders, technology suppliers, and research universities. Because of this, their clients may make use of the most cutting-edge AI technology currently accessible.
Avenga stands apart due to its dedication to AI research and development. They support their AI specialists in developing their careers by giving them access to regular training, research opportunities, and collaborative spaces.
Avenga’s commitment to staying on the cutting edge of AI means the company always has the knowledge to provide cutting-edge services that boost its clients’ bottom lines.
Headquarters: Warszawa, Poland
Founded: 2019
Size: 1000-10000
6. LeewayHertz
LeewayHertz has been in business since 2007, and it focuses on many areas of AI, such as ML, NLP, and computer vision. Big companies like Procter & Gamble, McKinsey & Company, Siemens, and Hershey’s are among its clients.
With the goal of helping organizations use AI on a large scale, LeewayHertz has made it onto our list of the Best AI Consulting Services companies because it provides innovative AI solutions across industries.
ML, speech recognition, and NLP were used to make Arya, the world’s first tea-making robot, in collaboration with an Indian food tech company. This was one of its most important projects. They have also made a store for AI apps that use computer vision and an employee time-tracking app that uses AI.
Headquarters: San Francisco, California
Founded: 2007
Rates: $50-$99 /hr
Size: 51-249
7. Azati
Azati was started in 2001. Its offices are in the US, and its research and development center is in Belarus. Both have helped many businesses, and the company has become one of the Best AI Consulting Services companies.
Depending on the needs of your project, the company has provided advice and built AI-powered solutions for both startups and large businesses.
They also offer design, programming, data science, and machine learning as part of their services. Azati has Top AI Development Experts to meet your goal of AI Data solutions.
Headquarters: Livingston, NJ, USA
Founded: 2001
Rates: $25-$49 /hr
Size: 100-145
8. Markovate
Markovate is a prominent AI development consulting firm that serves clients in a wide range of sectors with cutting-edge, tailor-made AI-powered solutions. Markovate has established itself as a leader in the artificial intelligence (AI) market by consistently releasing superior AI products that improve efficiency, delight customers, and fuel growth.
When it comes to technology and new ideas, Markovate is dedicated to being at the forefront among top artificial intelligence companies. They stay abreast of developments in artificial intelligence so that they may offer clients innovative strategies that provide results.
Markovate recognizes the need for teamwork and open lines of communication for completing AI projects. They put a lot of effort into making long-term connections with clients so that they can be a trusted partner throughout the whole process of developing AI.
Headquarters: San Francisco, USA
Founded: 2015
Size: 53-105
9. SoluLab
SoluLab is an Expert AI Consulting Services firm and a service provider for decentralized software development. It is known for providing new solutions to businesses in many different industries.
SoluLab has a team of Top AI Development Experts with a lot of experience in machine learning, natural language processing, and computer vision, among other things. Their success comes from making AI plans that are specific to each client’s goals and helping them reach those goals.
SoluLab has done some important projects, like putting AI-driven personalization tools into place for an e-commerce business, which led to higher conversion rates and more satisfied clients.
SoluLab’s method of putting AI to use is all-encompassing and team-based. They put ethical AI practices, following rules, and sharing information at the top of their list so that clients can use AI technologies successfully.
Headquarters: Los Angeles, CA, USA
Founded: 2014
Size: 50-249
10. ThirdEyeData
ThirdEyeData is a well-known AI Data Solution and Expert AI Consulting Services company. They offer data strategy, predictive analytics, machine learning, and NLP. ThirdEyeData helps organizations use data to make smart decisions and succeed with their AI expertise.
ThirdEyeData’s AI implementations and industry contributions are noteworthy. They solved tough business problems for varied clientele across sectors. Their knowledge has increased operational efficiency, customer satisfaction, and client income.
ThirdEyeData prioritizes customer happiness. They focus on clients’ needs and goals. ThirdEyeData supports AI consultancy throughout the process. AI consultancy companies trust them because of their client satisfaction.
Headquarters: San Jose, CA, US
Founded: 2010
Size: 50-249
At Data-Nectar, we are committed to providing top-notch AI consulting services, US-based AI cloud service providers, and innovative generative AI solutions that will propel your business into the future. Contact us today to explore your AI experience.
Final Words on Best AI Consulting Services Company
Organizations must carefully analyze their needs and choose the best AI consulting Services partner as demand develops. This blog features 2023’s top Expert AI Consulting Services businesses, but further research and involvement are needed to make final judgments.
In conclusion, AI consulting firms help enterprises use AI and flourish sustainably in the digital world. Businesses may stay ahead in the AI-driven age by using these top AI consulting firms.
Recent Post
Accelerate Data Workflows: Optimize Omnichannel sales with Delta Cache and Skipping
What is Ad Hoc Analysis and Reporting?
[pac_divi_table_of_contents included_headings="on|on|on|off|off|off" minimum_number_of_headings="6" scroll_speed="8500ms" level_markers_1="decimal" level_markers_3="none" title_container_bg_color="#004274" _builder_version="4.22.2" _module_preset="default"...
Top Benefits of Data Governance for Your Organization
[pac_divi_table_of_contents included_headings="on|on|on|off|off|off" minimum_number_of_headings="6" scroll_speed="8500ms" level_markers_1="decimal" level_markers_3="none" title_container_bg_color="#004274" admin_label="Table Of Contents Maker"...