[pac_divi_table_of_contents included_headings="on|on|on|off|off|off" minimum_number_of_headings="6" scroll_speed="8500ms" level_markers_1="decimal" level_markers_3="none" title_container_bg_color="#004274" admin_label="Table Of Contents Maker"...
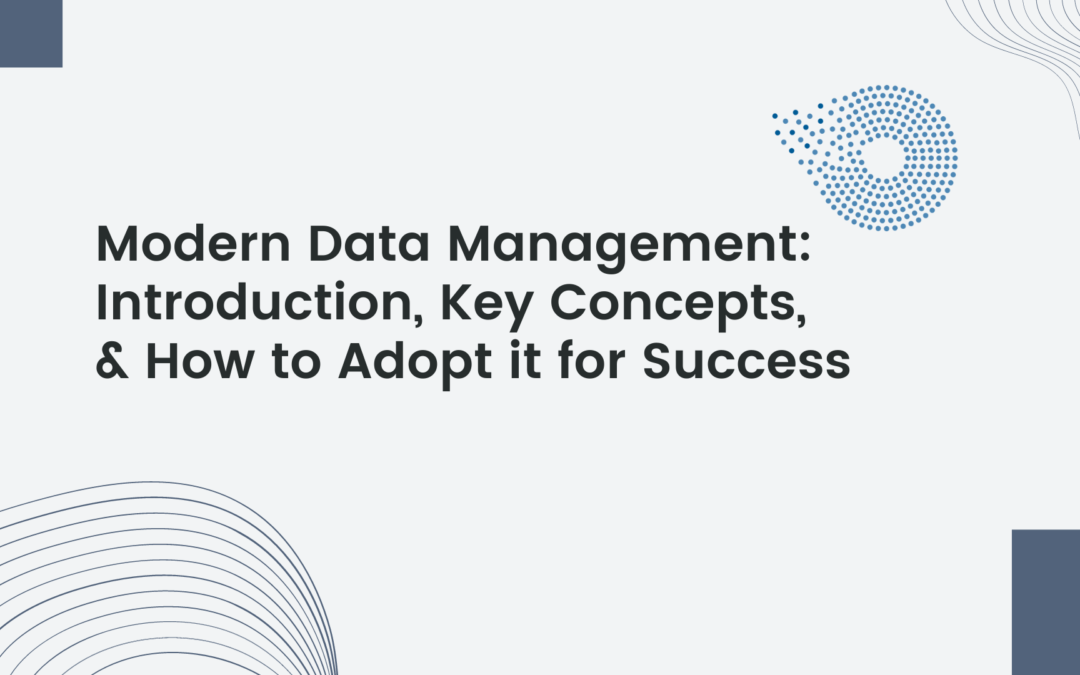
Modern Data Management: Introduction, Key Concepts, and How to Adopt it for Success
Data governance, integration, architecture, quality, and security are just a few aspects of modern data platform management essential to realizing the value of data inside an organization.
Given the sheer number of variables at play, it’s hardly surprising that businesses need help updating their data procedures.
However, you must carefully control every moving part of the machine. Newer technologies like automation and data modeling can help your company optimize its data management procedures and clarify common data problems.
What is Data Management?
Data management is a collection of multiple characteristics that, when taken together, allow businesses to use accurate and dependable data to generate insights that assist with decision-making. If a company wants to take a data-driven approach and rely on business intelligence tools to provide relevant reports, it must have a complete data management plan.
To guarantee that the enterprise’s data management strategy aligns with its objectives, policies and best practices must be developed and implemented across the whole company. The data management system is comprised of several different kinds of procedures, including:
- Data storage in the cloud and on-premises.
- Updating data in multiple data tiers.
- Security and recovery.
- Archiving historical data and eliminating it on schedule.
What is modern data management?
Modern data platform management is connecting data with technological advances to recognize possibilities and get insights to put it in the simplest terms possible. It lets businesses make choices more quickly and more effectively, which ultimately assists them in streamlining conventional data operations to gain a competitive edge.
Effective data management has become one of the most important challenges facing modern businesses due to the ever-increasing complexity of the new digital world.
Data Management: Why Do You Need It?
Many factors necessitate that a company prioritize data management and check for errors often. Businesses nowadays are increasingly turning to big data consulting services to help them modernize their operations and eliminate errors. It simplifies the changes and enhances the effects.
Data management risks and problems
Effective data management is crucial in today’s information-driven world, yet it comes with its fair share of risks and challenges. From data security breaches and compliance issues to data quality concerns and scalability problems, navigating the data landscape demands a strategic approach.
1) Safety of Information
The possibility of data breaches, illegal access, and cyberattacks all give substantial problems to data management. Broad security measures and constant monitoring are required to mitigate potential dangers and protect sensitive data.
2) Data Quality
In data management, one challenge is ensuring that the data are accurate, consistent, and extensive. Data that is inaccurate or inconsistent can lead to incorrect insights, which in turn can lead to poor decision-making and inefficiencies in processes.
3) Data Governance
Establishing and sustaining good data governance rules over time might be difficult. A concerted effort and a commitment on the company’s part are required to define data ownership, roles, and responsibilities, in addition to implementing data policies and standards.
4) Privacy and Compliance
It might be difficult to manage data in a way that complies with privacy requirements. Organizations are required to manage regulatory requirements, establish privacy measures, and handle data subject rights, all while keeping up to speed with the many rules that are continuously changing.
5) Data Integrability
The difficulty of integrating and combining data from various sources is a typical one. Engaging in careful preparation, data mapping, and integration strategy is necessary to achieve data interoperability between different systems, applications, and platforms.
6) Scalability and Volume
Managing and analyzing huge quantities of data gets more challenging as the volume of data continues to expand. To keep up with the ever-increasing need for data, you will need an infrastructure capable of scaling, effective storage, and data processing capabilities.
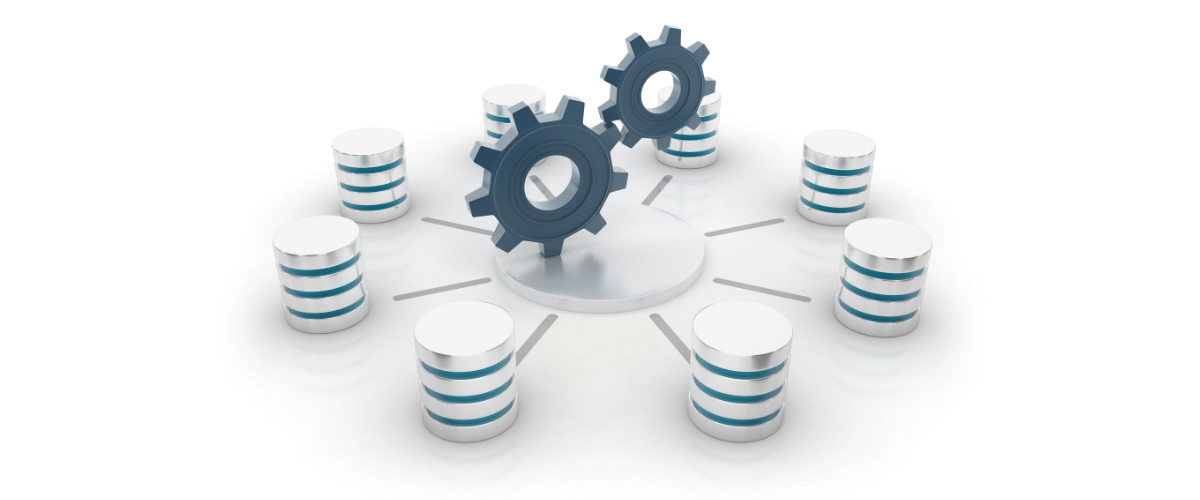
Benefits of Modern Data Management
The following is a list of benefits of modern data that an organization will receive if they use current data management strategies, regardless of whether they use on-premises or cloud-based services for data analytics.
1) Improved judgment
For decision-makers to make educated, data-driven choices, they need access to accurate, trustworthy, and up-to-date data.
2) Resource Minimization
If data scientists spend more than half their time just gathering and organizing information, they waste their time. When will there be an analysis of this data? Do we not have access to real-time information? The solution is found in the use of modern data management strategies and consulting services.
Businesses may maximize their profits by investing in AI-based technologies, advanced software for data analytics, and automating routine operations.
3) Safety and reliability of data
To improve the dependability and integrity of data assets, data management strategies center on keeping data quality high by guaranteeing its correctness, consistency, and completeness.
4) Insights and customization
The ability to collect and analyze client data is made possible by efficient data management, allowing businesses to get insights that can be utilized to tailor their services, boost customer happiness, and win their loyalty.
5) Save money
Through better modern data strategy, businesses may save money by reducing unnecessary data duplication, saving money on storage and infrastructure, and avoiding data-related mistakes.
6) Privacy and data security
The potential for data breaches and negative loss may be reduced by putting in place data management procedures that keep data safe, prevent unauthorized access, and comply with the requirements for privacy.
7) Recovering from disasters
The ability to recover from data loss or system outages is important, which is why disaster recovery plans are integral to data management.
8) Data and collaboration
With the right modern data strategy, teams and departments can easily share and collaborate on data to improve workflow, communication, and cross-functional understanding.
Data Management Tools and Technologies
Data management tools and technologies are built on platforms for managing data and feature a variety of components and procedures that function together to help you get the most out of your data. Database management systems, data warehouses and lakes, data integration tools, analytics, and other tools fall into this category.
Database management systems (DBMS)
There are several types of database management systems consisting of,
- Relational database management systems (RDBMS)
- Object-oriented database management systems (OODMBS)
- In-memory databases
- Columnar databases
What are data warehouses and lakes?
1) Data warehouse:
A data warehouse is a centralized collection of data from multiple sources for reporting and analysis.
2) Data lake:
A data lake is a large collection of raw or natural data. Data lakes are commonly used to store Big Data, which can be structured, unstructured, or semi-structured.
Master data management (MDM)
Master data management is establishing a single trustworthy master reference for all critical company data, such as product, customer, asset, finance, and so on.
MDM ensures that enterprises do not employ numerous, potentially conflicting versions of data in various sections of the business, such as processes, operations, analytics, and reporting. Data consolidation, governance, and quality management are the three main pillars of efficient MDM.
1) Big Data management
New databases and technologies have been developed to manage Big Data – vast volumes of structured, unstructured, and semi-structured data inundating enterprises today.
New approaches to analyzing and managing data diversity have been developed, including highly effective processing techniques and cloud-based capabilities to handle the volume and velocity.
To enable data management technologies to understand and interact with various types of unstructured data, new pre-processing procedures, for example, are employed to recognize and categorize data items to facilitate storage and recovery.
2) Data integration
The practice of absorbing, manipulating, merging, and delivering data where and when it is required is known as data integration.
This integration occurs within and outside the organization, spanning partners, third-party information sources, and use cases to meet the data consumption requirements of all applications and business processes.
Bulk/batch data transfer, extract, transform, load (ETL), change data capture, data replication, data visualization, streaming data integration, data orchestration, and other techniques are used.
3) Data governance, security, and compliance
Data governance is a set of policies and responsibilities that ensure data availability, quality, compliance, and security within an organization.
Data governance sets up the systems and identifies the individuals inside an organization who have power and responsibility for the processing and security of various types of data.
Data governance is a critical component of compliance. The technology will handle the technical aspects of storage, handling, and security.
It includes the people side and the governance side, which ensures that the data is correct, to begin with and that it is managed and secured properly before being entered into the system, while it is being used, and when it is extracted from the system for use or storage elsewhere.
Governance defines how accountable persons use processes and technologies to manage and secure data.
Of course, in today’s age of hackers, viruses, cyberattacks, and data breaches, data security is a huge worry.
While security is incorporated into systems and applications, data governance ensures that those platforms are correctly set up and managed to protect the data and that procedures and responsibilities to safeguard the data outside the systems and database are followed.
4) Analytics and business intelligence
Data management systems contain basic data collection and reporting features, and many contain or package developed retrieval, analysis, and reporting applications.
Third-party developers offer reporting and analytics applications, which will virtually probably be part of the application bundle as an integral part or as an extra module for more extensive capabilities.
The power of current data management systems is largely derived from random retrieval capabilities, which enable users with no training to design their on-screen data retrievals and print-out reports with unexpected formatting, calculations, sorts, and summaries.
Professionals can also use these tools or more powerful analytics tools to perform more calculations, comparisons, higher math, and formatting. New analytics apps may connect traditional databases, data warehouses, and data lakes, combining Big Data with business application data for better forecasting, analysis, and planning.
What Is An Enterprise Data Strategy, And Why Do You Need One?
With today’s data explosion and its importance to the operation of every company, a more proactive and complete approach to data management is becoming increasingly required.
In practice, this involves preparing ahead of time and developing a modern data strategy that:
- Identifies the exact categories of data that your organization will require and utilize
- Assigns responsibility for each type of data
- Creates policies to govern the gathering, collection, and use of that data
Wrapping It Up
Maintaining modern databases requires a multidisciplinary approach that incorporates several different procedures, technologies, and personnel. A company cannot successfully implement the contemporary data management method unless these are consolidated onto a single platform. Many data management businesses counsel small and large businesses on the need to adopt such a system and how to most effectively put it into practice.
It’s time to switch to a data-driven strategy so the company can grow and thrive in today’s increasingly competitive business climate.
Recent Post
Top Benefits of Data Governance for Your Organization
Enhancing Customer Engagement with Data Management Platforms and Omni-Channel Strategies
[pac_divi_table_of_contents included_headings="on|on|on|off|off|off" minimum_number_of_headings="6" scroll_speed="8500ms" level_markers_1="decimal" level_markers_3="none" title_container_bg_color="#004274" admin_label="Table Of Contents Maker"...
AI Personalization Excellence: Enhancing Customer Engagement in 2024
[pac_divi_table_of_contents included_headings="off|on|on|off|off|off" scroll_speed="8500ms" level_markers_3="none" title_container_bg_color="#004274" _builder_version="4.22.2" _module_preset="default" vertical_offset_tablet="0" horizontal_offset_tablet="0"...