[pac_divi_table_of_contents included_headings="on|on|on|off|off|off" minimum_number_of_headings="6" scroll_speed="8500ms" level_markers_1="decimal" level_markers_3="none" title_container_bg_color="#004274" admin_label="Table Of Contents Maker"...
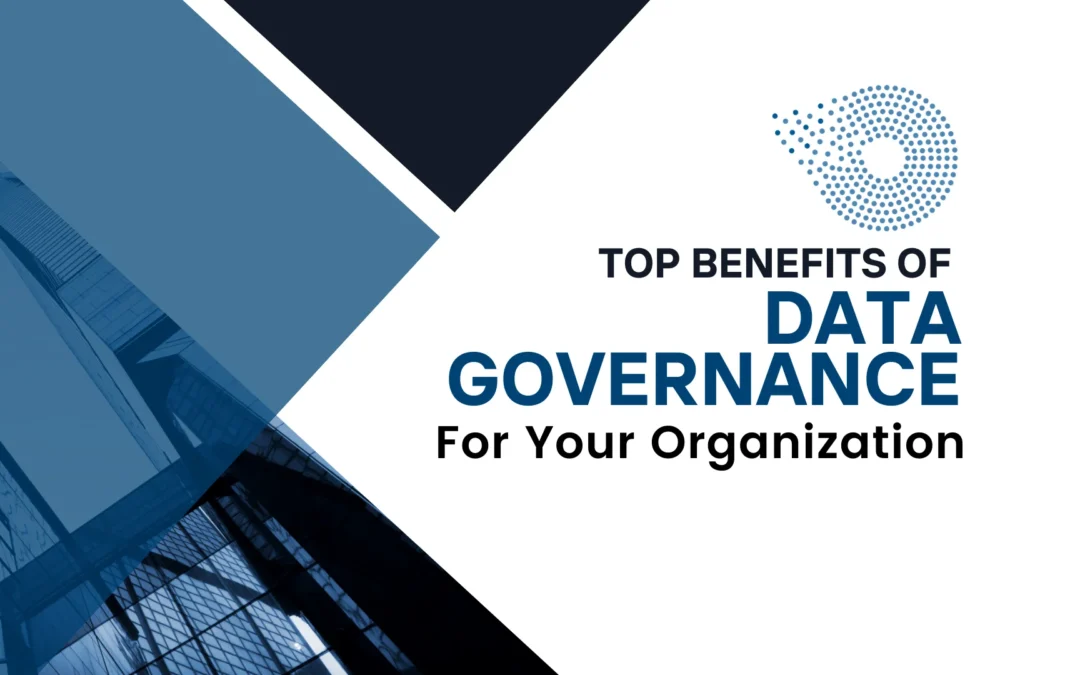
Top Benefits of Data Governance for Your Organization
In today’s data-driven world, organizations of all types and sizes generate vast amounts of data daily. This data, if managed correctly, can be a powerful asset, driving informed decision-making, enhancing operational efficiency, and providing competitive advantages. However, to harness this potential, robust data governance practices must be in place. In this blog post, we will explore what data governance is, its main role, goals, importance, and benefits. By the end, you will have a clear understanding of why effective data governance is essential for your organization.
What is Data Governance?
Data governance is the process of managing the availability, usability, integrity, and security of data used in an organization. It involves a set of policies, procedures, and standards that ensure data is consistently handled and maintained across the enterprise. The primary aim is to ensure that data is accurate, reliable, and accessible while safeguarding it from misuse and breaches.
What are The Main Role of Data Governance
The main role of data governance is to establish a framework for data management that aligns with the organization’s goals and regulatory requirements. This framework includes defining data ownership, data quality standards, data security protocols, and compliance measures. Key roles within data governance typically involve data stewards, data owners, and data governance committees.
- Data Stewards: These are individuals responsible for the management and oversight of specific data domains within the organization. They ensure data policies are followed and act as a bridge between technical and business aspects of data management.
- Data Owners: Data owners are accountable for the data within their respective areas. They make decisions about who can access the data and how it should be used.
- Data Governance Committee: This committee is responsible for establishing and enforcing data governance policies. It typically includes representatives from various departments to ensure a holistic approach to data management.
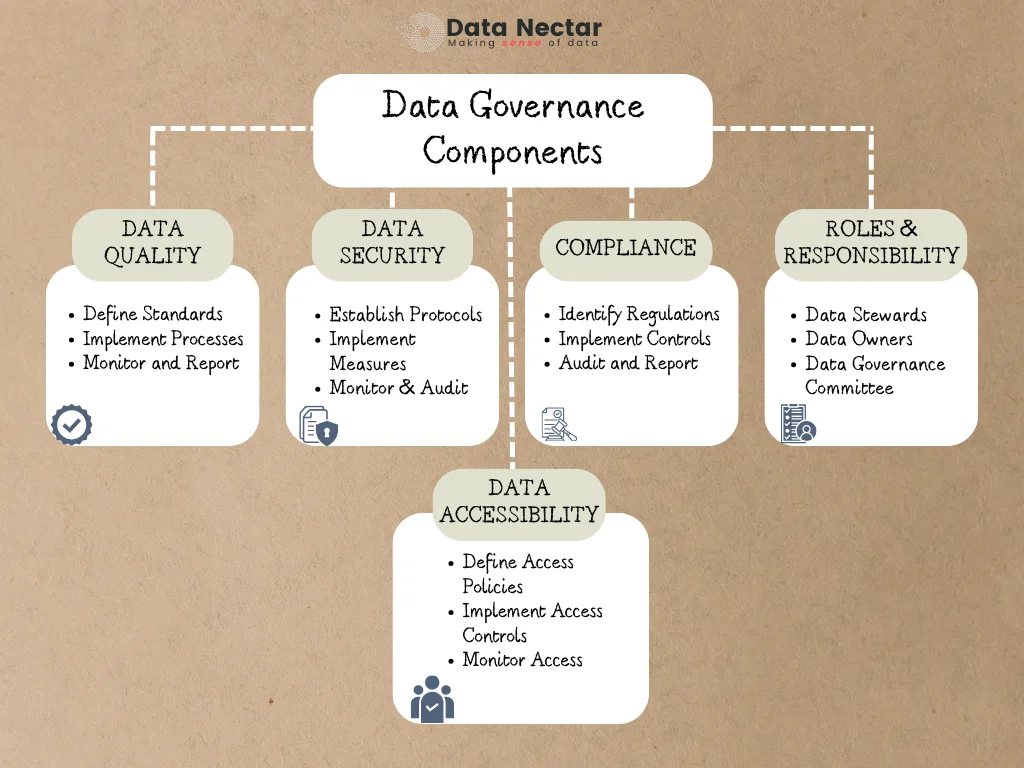
What is The Goal of Data Governance?
The primary goal of data governance is to ensure that data is treated as a valuable asset. This involves:
- Ensuring Data Quality: Implementing standards and procedures to maintain the accuracy, completeness, and reliability of data.
- Enhancing Data Security: Protecting data from unauthorized access, breaches, and other security threats.
- Improving Data Accessibility: Making sure that relevant and accurate data is easily accessible to those who need it within the organization.
- Ensuring Regulatory Compliance: Adhering to legal and regulatory requirements related to data privacy and security.
Why its Important?
Effective data governance is crucial for several reasons:
- Decision-Making: Reliable and high-quality data is essential for making informed business decisions. Without proper governance, data can be inconsistent, inaccurate, or incomplete, leading to poor decision-making.
- Regulatory Compliance: Many industries are subject to stringent regulations regarding data privacy and security. Effective data governance ensures that organizations comply with these regulations, avoiding legal penalties and protecting their reputation.
- Operational Efficiency: Proper data governance streamlines data management processes, reducing redundancy and improving efficiency. This can lead to cost savings and better resource allocation.
- Risk Management: Data governance helps identify and mitigate risks associated with data management, including data breaches and misuse. This protects the organization from potential financial and reputational damage.
- Customer Trust: In today’s digital age, customers are increasingly concerned about how their data is used and protected. Effective data governance helps build and maintain customer trust by ensuring data is handled responsibly and transparently.
The Main Benefits of Data Governance
Implementing a robust data governance framework offers numerous benefits to organizations:
- Improved Data Quality: Ensuring that data is accurate, consistent, and reliable enhances its value and utility.
- Enhanced Security: Strong data governance policies protect sensitive data from breaches and unauthorized access, safeguarding the organization’s assets.
- Regulatory Compliance: Effective data governance ensures compliance with relevant laws and regulations, reducing the risk of legal issues and fines.
- Better Decision-Making: High-quality data supports better strategic and operational decision-making, driving business growth and success.
- Increased Efficiency: Streamlined data management processes reduce duplication of effort and enhance overall operational efficiency.
- Risk Mitigation: Identifying and addressing data management risks proactively protects the organization from potential threats.
- Customer Trust and Satisfaction: Transparent and responsible data practices build customer trust and enhance the organization’s reputation.
At Data-Nectar, we understand the critical role data governance plays in driving business success. Our expert team is dedicated to helping you implement effective data governance practices tailored to your organization’s unique needs. Contact us today to learn how we can support your data governance journey and unlock the full potential of your data.
Conclusion
Data governance is more than just a buzzword; it’s a fundamental practice that ensures the integrity, security, and usability of your organization’s data. By understanding its roles, goals, and benefits, you can implement a robust data governance framework that drives informed decision-making, enhances operational efficiency, and builds customer trust. Start your data governance journey with Data-Nectar today and transform your data into a powerful asset for your business.
Implement Effective Data Governance Today
Implement effective data governance with Data-Nectar’s expert solutions. Contact us for tailored data governance practices.
Recent Post
Top Benefits of Data Governance for Your Organization
Enhancing Customer Engagement with Data Management Platforms and Omni-Channel Strategies
[pac_divi_table_of_contents included_headings="on|on|on|off|off|off" minimum_number_of_headings="6" scroll_speed="8500ms" level_markers_1="decimal" level_markers_3="none" title_container_bg_color="#004274" admin_label="Table Of Contents Maker"...
AI Personalization Excellence: Enhancing Customer Engagement in 2024
[pac_divi_table_of_contents included_headings="off|on|on|off|off|off" scroll_speed="8500ms" level_markers_3="none" title_container_bg_color="#004274" _builder_version="4.22.2" _module_preset="default" vertical_offset_tablet="0" horizontal_offset_tablet="0"...